What is Data-as-a-Product?
- Kaycee Lai
- Jul 11, 2023
- 4 min read
Better data access improves business performance, but it also requires significant changes in our approach to data architecture. ‘Data-as-a-Product’ presents a paradigm shift in how organizations manage data to make data democratization a reality.
Put simply, Data-as-a-Product means delivering data in a way that allows your company’s users, at all skill levels, to get immediate value.
The concept is gaining traction with the emergence of defined approaches like the data mesh, pioneered by Zhamak Dehghani of ThoughtWorks, who proposed a methodology to:
Address rapidly growing data volumes and use cases
Enable quick access to datasets across a large organization
Address shortcomings of traditional centralized data architecture, including those of data warehouses and data lakes
Relieve administrative burden on central data teams, while empowering business units with easy access to data insights
Data-as-a-Product achieves these objectives by creating a customer-centric product mindset around organizational data.
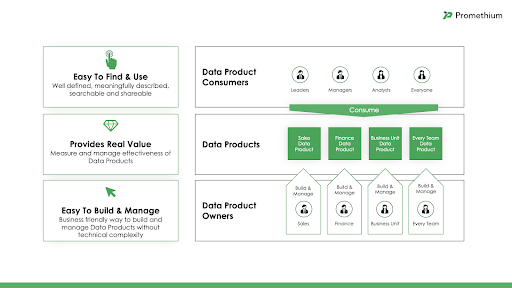
Making your data ‘product’ appeal to your customer audience
Successful product lines are tailored to specific customer needs. For data products the customer list includes anyone who needs data, ranging from data engineers, data scientists and analysts, to business users who lack technical expertise.
Let’s explore some critical elements that make data products suitable for this customer base:
Easy to Find and Use. Relying on SQL to retrieve datasets prevents most users from getting answers. For data to be productized, NLP must be leveraged to allow people to search for datasets in Google-fashion. Furthermore, the information retrieved about the data must be as well-defined, meaningfully described, and as shareable as a Google snippet.
Department-specific Value. Data must be productized to meet department-level needs, being configured for sales, finance, marketing, and other areas.
Easy to Build & Manage. Questions from different departments often require the same datasets. Furthermore, many require ‘federated’ data combined from multiple sources. The old-school route for this involves moving data from different repositories into a central data warehouse. Productizing data, however, requires the ability to join datasets from different sources without technical complexity, and without having to move any data.
Easy to Consume. For data to be quickly used it must be delivered through apps that allow even non-technical users to easily visualize it, collaborate, and tell stories.
Easy and Secure Organization-wide access. Even if the right data is located, it’s useless if it can't be quickly accessed. Data-as-a-Product involves streamlining user access processes with governance that supports the organization without slowing it.
Easy to Measure and Monitor. What successful product line is maintained without a customer feedback loop? You must continually monitor usage and gauge effectiveness, collecting such metrics as the number of queries a data product receives, and the number of answers it yields.
Data-as-a-Product is Like Apps on a Smartphone
To better understand Data-as-a-Product, think about how its core principles mirror something we use daily--smartphone apps. It’s difficult to imagine life without our various ‘exchanges’, which deliver apps that:
Are easily searchable in one convenient place
Are easy to consume
Have clearly designated owners
Provide descriptions to help you quickly decide whether they meet your needs
Give you the ability to rate their effectiveness
These principles are in full force in the Data-as-a-Product paradigm. Let’s say you’re in the marketing department of a large enterprise and have a question that requires data. The traditional way might involve passing the question along to the central data team, who serve as a kind of customer support for data. From there it disappears into a black box and when the answer finally emerges it’s too late to be actionable.
Contrast this support ticket approach to the Data-as-a-Product way which, like finding a smartphone app, involves connecting to a central platform, conducting a Google-like search in natural language on your business problem, and getting:
A selection of data products along with easy-to-understand descriptions
History on how they’ve been used in the past that helps you understand if it is fit for your purpose
The end result is a curated and prepped dataset, visualized through user-friendly tools that allow you to quickly dive in and get answers. This begs the question, who is responsible for getting data into this form?
The Ongoing Job of the Data Product Manager
With the old way of doing things each data dive is a one-off project. You find the data, get what you need, and move on. Data-as-a-Product, however, assumes that you’re not the first to ask a question like this, nor will you be the last. Under this approach a data product is ongoing, with continual improvements driven by a customer feedback loop.
Furthermore, the idea that data is an ongoing product implies a product owner with responsibility for overseeing its development. Consider, for example, a major financial services provider I recently spoke with that wants to implement a model with a “data product manager” for each business unit, who is empowered to:
Know what data their business unit needs and where to find it
Identify what data can be used
Quickly assemble data from different parts of the organization
Collaborate on the data product with subject matter experts within their own department, and members of the central data team
Note that this person is not part of the organization’s central data team, but rather someone operating at the department level. For example, the data owner for marketing-related data products would be someone on the marketing team. The ability of someone who is not a data engineer or data scientist to take on data product ownership is made possible by some of the previously mentioned features of Data-as-a-Product, such as ease of finding data, and ease of building and managing data.
Delivering Speed, Productivity and Opportunities
With a Data-as-a-Product mindset and customer-ready data products in place, expect such improvements as:
Speed and agility, with reduced wait time for data
Fewer missed opportunities
Increased productivity and reduced skill debt with data engineers and scientists spending their time on innovation, rather than soon-to-be obsolete processes
In sum, Data-as-a-Product brings a simultaneous increase in ROI and lower TCO for analytics.