Repost of the original article on AI TechPark
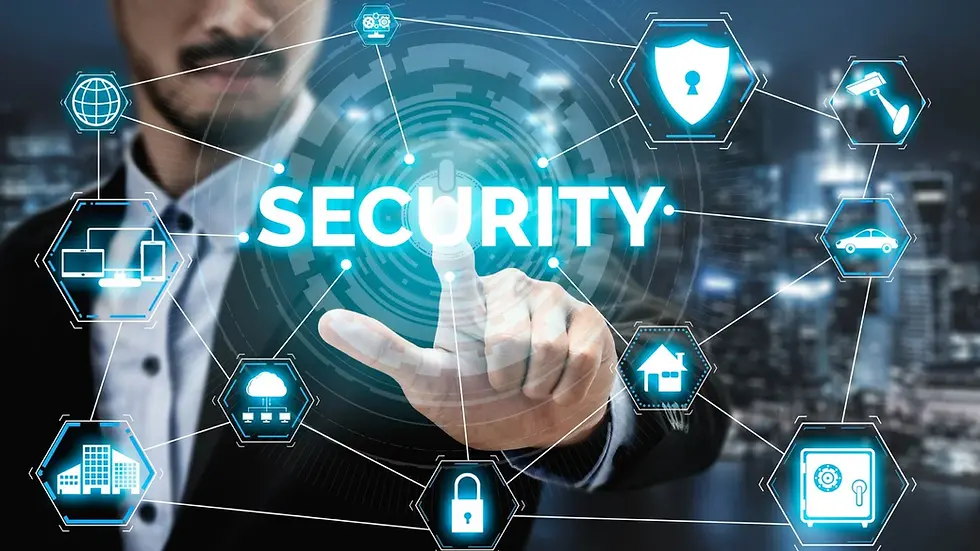
Master enterprise security with GenAI. Learn how data fabrics and active metadata redefine governance and safeguard sensitive data.
Generative AI (GenAI) has the power to revolutionize how enterprises operate by enabling new levels of automation, efficiency, and innovation. However, implementing GenAI presents multiple challenges, including data privacy and security. According to Gartner’s Generative AI 2024 Planning Survey, data protection and privacy sit among the top of the list, being named as a key concern by 39% of data and analytics leaders. But what exactly is driving these challenges? Traditional data management approaches, which rely on fragmented data sources and siloed governance protocols, are increasingly inadequate in today’s era of Large Language Models (LLMs). Current methods are falling short and leading many to explore modern approaches, such as the data fabric, to address these security and governance concerns effectively.
The reason is that enterprises have historically managed data across various sources and storage systems, each governed by distinct security protocols and policies. While manageable in simpler data environments, this model becomes problematic when deploying LLMs, as they require access to diverse and extensive datasets to function effectively. The traditional siloed approach makes it challenging to integrate these disparate data sources seamlessly, leading to inefficiencies and potential security gaps. It also makes training and fine-tuning LLMs much more complicated, as point solutions lack the breadth necessary to provide holistic context to LLMs.
As a result, the traditional approach often necessitates either consolidating every piece of data in a single warehouse – which is time-consuming, expensive, and inefficient – or sending data to public or external LLMs. However, this practice can expose sensitive information, leading to potential security breaches and compliance violations. Given these challenges, enterprises must adopt a more cohesive and secure data management strategy to harness the full potential of GenAI without compromising security and governance.
How a Data Fabric & Active Metadata Enhance Security and Governance
A data fabric offers a comprehensive solution to the security and governance challenges posed by integrating GenAI into enterprise environments. By providing a unified and intelligent data management layer, a data fabric addresses the key concerns effectively by providing an abstraction layer between data and LLMs, leveraging active metadata for secure prompt engineering, and providing a single API for metadata access.
One of the foremost security concerns with deploying LLMs is the risk of exposing sensitive data to the public. A data fabric mitigates this risk by acting as an intermediary layer that ensures sensitive data is never directly sent to the LLM. Instead, the data fabric manages data access and retrieval, allowing the LLM to interact with data in a controlled and secure manner. This approach prevents unauthorized access and reduces the risk of data breaches, as the LLM only processes the information necessary for generating responses without handling raw sensitive data.
Active metadata plays a crucial role in enhancing security and governance within a data fabric. By applying machine learning to metadata, it is transformed into actionable insights that guide how data is accessed and utilized. This capability is vital for secure prompt engineering, where prompts to the LLM are crafted based on metadata rather than direct data access. Key aspects of metadata include data lineage and provenance, quality metrics, or usage statistics. By tracking data access and usage, active metadata helps manage privacy and security risks, ensuring compliance with regulatory requirements. Through these mechanisms, active metadata ensures that interactions with LLMs are secure, governed, and compliant with organizational policies.
A data fabric centralizes access through a single API, streamlining interactions between LLMs and the organization’s data landscape. Instead of connecting to multiple data sources with varying protocols and security measures, the LLM interacts with a single API that provides unified data access. This simplification reduces complexity and the potential for security vulnerabilities. By exposing only metadata and not the underlying data sources, the data fabric ensures that the LLM does not have direct access to sensitive data. Additionally, this abstraction layer enforces consistent security policies and access controls across all data interactions. A single API also ensures that governance policies are uniformly applied, preventing inconsistencies and ensuring compliance with data protection regulations. As enterprises increasingly adopt Generative AI, ensuring strong, reliable data security and governance becomes paramount. Traditional data management approaches, with their fragmented and siloed structures, are ill-suited to meet the demands of integrating today’s LLMs effectively and securely. Adopting a data fabric offers a scalable and secure framework that addresses these challenges by ensuring no data is sent directly to LLMs, leveraging active metadata for secure prompt engineering, and providing a single API for metadata access without exposing underlying data sources.